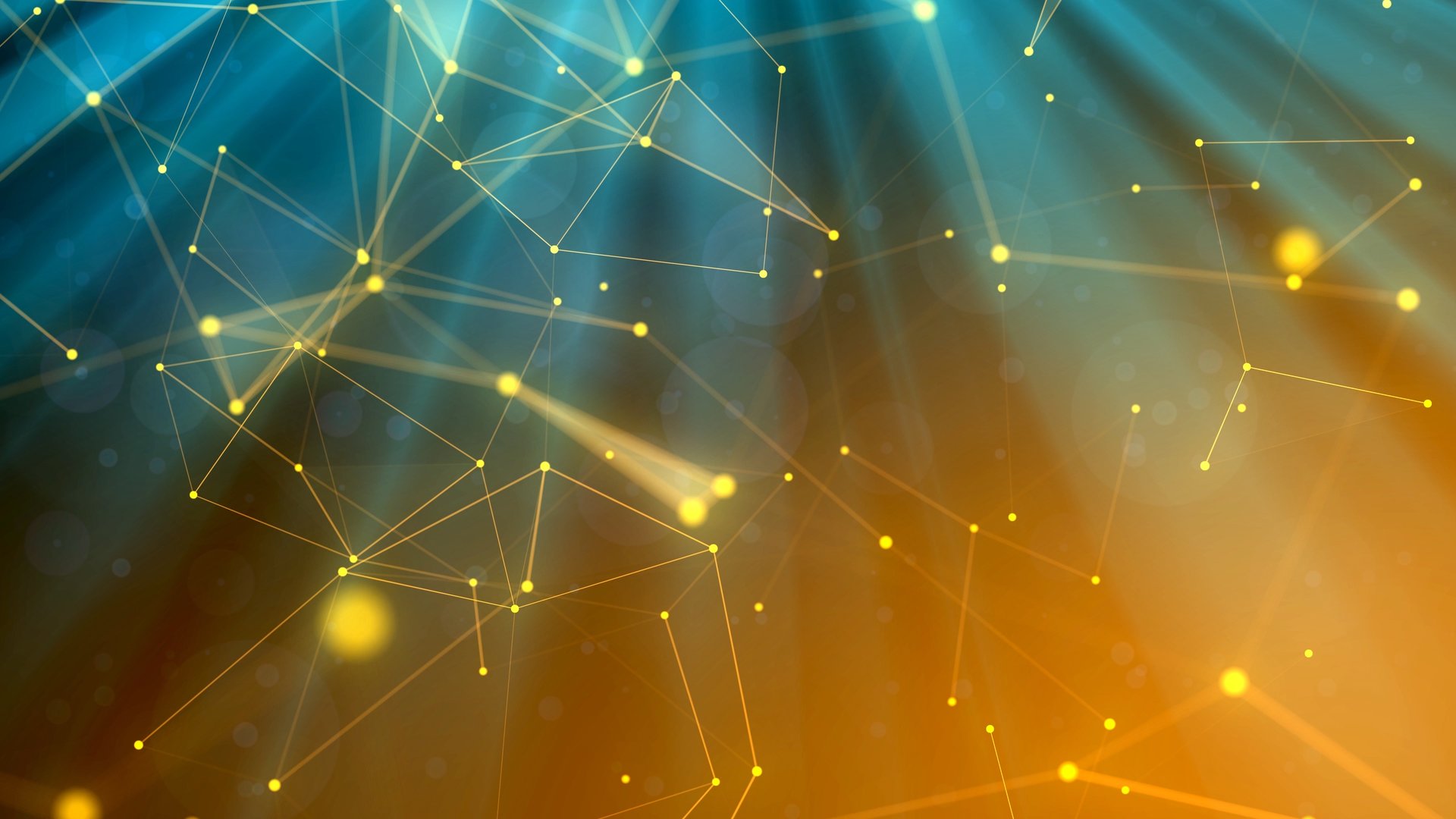
The Pheno-Type
Calum MacRae on his “hopelessly diffuse” career in science and medicine
“The Pheno-Type” takes a closer look at the people behind phenomics-driven drug discovery.
Calum MacRae is a cardiologist, geneticist, developmental biologist, and longtime scientific advisor to BioSymetrics. He is the Vice Chair for Scientific Innovation at the Department of Medicine at Brigham and Women’s Hospital and Professor of Medicine at Harvard Medical School. In addition, Dr. MacRae is a Principal Faculty Member at the Harvard Stem Cell Institute and an Associate Member at the Broad Institute. Below we interview Calum about his journey to become a physician-scientist and what excites him about the future of R&D.
Calum MacRae, MD, PhD
BioSymetrics Advisor
Q: How did you become the physician-scientist that you are today?
Calum: I’ve always been interested in science and medicine. I am a seventh-generation physician. My father was one of only a few primary care physicians on the island where we lived growing up. As a young child, people would come to our house to receive care; the clinic was our living room.
I became attracted to fundamental science as an undergraduate, where I did work on the basic physiology of kidney disease. Through that experience and my training in medicine, I started to learn how we oversimplify the classification of patients. Patients that had very different outcomes were being lumped together in big, generic groups. This was largely a function of the tools we had to measure outcomes. You can only recognize patterns as being different if you can measure those differences.
That got me thinking about how to analyze people at a higher resolution. When I moved to Boston to work in genetics, on inherited heart disease, I was struck by how we were analyzing patterns one family at a time, one disease at a time. I wondered if there were technologies that would allow us to analyze biology at a higher-resolution and in higher-throughput. This is when I began to learn more about the engineering principles that wire underlying biology.
Working at Harvard, I met Mark Fishman (who would later become the CSO of Novartis). He presented the outcomes of a genetic screen for heart mutants in zebrafish, which changed my whole view of what was possible in genetic research. I went to Massachusetts General Hospital to study the fundamental mechanisms of heart disease and continued my work in zebrafish, looking at the developmental basis of human disease. I also began to devote my own lab to exploring how we might replicate this in humans. We started working on systematic approaches to patterns in genes that cause heart failure. The goal of our lab is to build techniques that would allow us to profile, systematically, the biological system that is the zebrafish, where everything is connected and wired as it should be. And then to connect that system to humans through both genes and phenotypes. In other words, a way to systematically connect human health and disease.
The last 20 years of my career has been spent focused on the next step in this convergence… phenotypic-driven screening for suppressors of disease. Can we collect more granular data on patient disease so that we can stratify people to see their entire life trajectory to health or disease? This systems approach to biology and medicine, linking the cellular and physiological in parallel in zebrafish and human, is now my career focus and gives me a lot of hope for applying scale to drug discovery, something simply not possible with other vertebrate model organisms today.
Q: As a research scientist, how does the experimentation model you use play a role in developing a systematic approach to discovering biology?
Calum: Ultimately, we need a broader repertoire of models, or series of model systems, that give you different levels of insight into different aspects of the biology or pharmacology. Zebrafish, which we use in my lab and BioSymetrics uses in their approach, when it was first developed as a model organism, was met with some skepticism. Some people thought why do we need another model system? Mouse was already very successful. One of the challenges with mouse is also one of its advantages; the extent of in-breeding which enables precise genetic manipulation but can also lead to less representative phenotypic outcomes. In addition, rodent metabolism and physiology is very far from that of other vertebrates and may not be a perfect representation of human disease. Similarly, yeast, fly and worm offer a ton of tractability but do not share enough commonality to humans. Zebrafish fits nicely in that middle ground. As we establish a broader repertoire of models, people will continue to see zebrafish as a powerful tool in mechanistic biology and drug discovery.
When looking at complex relationships between multiple genes, we can piece together a quantitative picture of how genes interact. Researchers are starting to realize the importance of these gene to gene and gene to environment interactions. That said, it’s not solely about the animal or experimental model… the data wrangling, cleaning and machine learning is as important, as you must be very comfortable with navigating diverse datasets.
“With the BioSymetrics approach, it is possible to build programs from the outset for the right targets and overcome many of the challenges in drug discovery that result in only 1 in 10 drugs making it to the clinic.”
Q: You’re clearly a busy person. Why dedicate time to advising BioSymetrics and what excites you about our approach?
Calum: BioSymetrics’ ability to move quickly and effortlessly between human and model datasets is differentiating. The tools that BioSymetrics is developing allow you to map from highly reproducible, basic biology datasets onto a clinical landscape and build end-to-end pipelines to continue mapping through the discovery science. That is what truly appeals to me. Essentially, you’ve created a systems approach to drug discovery, as opposed to a series of one-off experiments. When you make these maps at a high enough resolution, you start to reach the point at which you can begin to observe exponential insights.
Other companies in the same sector as BioSymetrics have not included scalable models capable of taking their AI-driven systems approaches into an in vivo setting. They are dependent on cell autonomous phenotypes. Unfortunately, many diseases are cell non-autonomous. The next phase then is… how do we map these cellular phenotypes into a whole animal. And that is a space in which BioSymetrics is already well positioned. One thing I’ve admired about BioSymetrics is the team’s ability to skate to where the puck will be… it can be hard to sell something that people are still realizing they need, but that excites me.
With the BioSymetrics approach, it is possible to build programs from the outset for the right targets and overcome many of the challenges in drug discovery that result in only 1 in 10 drugs making it to the clinic.
Q: Thank you for the encouraging words, Calum. Finally… as a person with a career that is at the convergence of science, healthcare and technology, what would you say to someone who is interested in exploring more than one discipline throughout their career?
Calum: A lot of scientific fields really are all converging. The rise of computing power has connected science and medicine in a way that we’re still at the very outset of realizing. For example, we are working with large datasets so that we can refine to a greater level of granularity the diseases we are trying to cure. Unfortunately, modern medicine is data sparse… even within the electronic medical record, most data are unstructured and of limited utility. So, we need to generate more and better data in the clinic and in our research models. My experience has taught me that it is at these interfaces that the challenges will lie for some time… and also the opportunities for innovation. This type of diffusion in my scientific work is a feature not a bug. I am hopelessly diffuse. And proud of it.
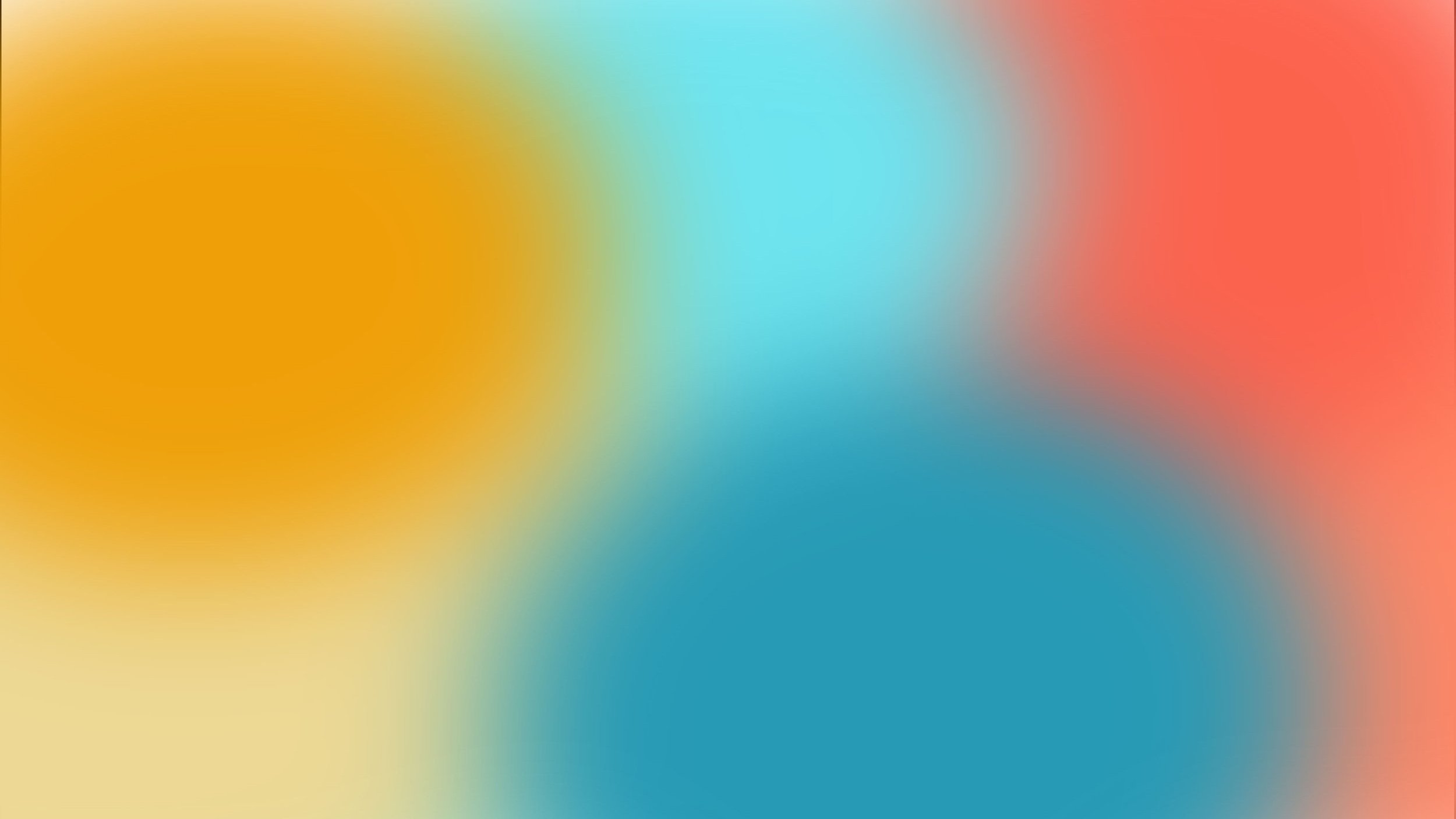